Here you will learn about how organizing data and employing data governance procedures will empower the data from primary activities of the value chain. And how valuable insights can be obtained through applied analytics.
1. INTRODUCTION
The motive of this whitepaper is to provide valuable insights about how Data Governance along with analytics can contribute to the value chain of manufacturing companies.
Descriptions of some terms which help you in understanding the discussion
- Value Chain in Business: It is a set of activities that a business executes to deliver a product or service for the market. Primary activities in the value chain include Supply chain management, Operations, Marketing and Sales, service, and support. Whereas the support activities include Technology, Research and Development, Procurement, Legal, Finances, and Human resource management.
- Data Governance: It is the way toward dealing with the accessibility, convenience, reliability, and security of the data in big business frameworks, in light of interior data norms and policies that likewise control data utilization. Successful information administration guarantees that information is steady and reliable and doesn’t get abused.
- Technical Metadata: It defines the information related to data which include- permissions, physical properties of the database, performance tuning, basic descriptives of the data, relations, constraints, etc.
- Business Metadata: When a set of rules is associated with data that adds value to it from a business perspective. For example, generating insights from metadata using statistical methods and visualizations
- Business Intelligence: It is an innovation-driven procedure for breaking down information and introducing noteworthy data that helps teams, managers, decision-makers and other corporate end-clients to settle on educated business decisions.
Poor organization and cleaning of data then managing it for relevant analysis are causing businesses a lot of their time and effort. Teams are facing difficulties in finding out the correct and reliable data. They spend a lot of their time doing the same set of redundant operations to prepare data for analyzing as per business needs. Consequently, it is impacting the productivity of Data Analysts and Data Scientists at the same time lower returns on investment.
These data-related issues usually arise due to many reasons and the majority of those are
- Poor data at the source
- Inexperience in data collection
- modifications in Business and operations like- Mergers, Acquisitions, Alliances, infrastructure transformation,
- Business expansion to new territories and the introduction of new products
- To resolve such issues, businesses start the accumulation of more raw data which further complicates the problem impacting value driven from data.
Data keeps on generating all the time from multiple sources as part of various business operations. There are 3 types of data sources
- Professional sources: Industry databases, archives, invoices, user logs, company sites, etc.,
- Contributed sources: User-generated data like reviews, experiences, recommendations, etc.
- Intentional sources: Data collected for enhanced user experience, Feedback data, Data used in primary research, Metadata, etc.
- The data from these sources get continuously accumulated over time. Data can be collected either through automated tools or manual queries.
2. GETTING STARTED: STEPS TO DATA MODERNIZATION
The journey to governing data at scale starts with the identification of data sources, developing a pipeline to extract data, cleaning and re-engineering data, curation, storage and ends with getting insights through prepared data models. We have distributed the entire journey, to getting analytical insights from raw data, into five major steps in the Fig.1. For each step, a respective set of activities is allocated. For instance, an organization that is into manufacturing probably look out for the following series of activities to achieve BI and analytics
- Source Identification All the relevant sources of data will be identified that includes devices, sites, invoices, data related procurements, distribution, manufacturing processes, Supply chain, ERP, local files and servers, etc. and the metrics will be studied to create a proof of concept
- Acquisition and Analysis Data will be acquired and re-engineered at scale using Stream mining and Machine learning procedures
- Curation Data validation and quality checks that eliminates any outliers or corrupted data. This step may require automated solutions and human computation at scale. Datasets will be created about the primary activities.
- Storage and management Data will be instantiated at a server or public/private cloud from all the connected sources in real-time. Data will be standardized at scale while maintaining a database with associated permissions. Relations among the datasets will be created with user authorizations
- Usage and Analytics Data from devices will be monitored in real-time through interactive dashboards. For instance, predictive models can be created to plan manufacturing activities based on the historic data from primary activities.
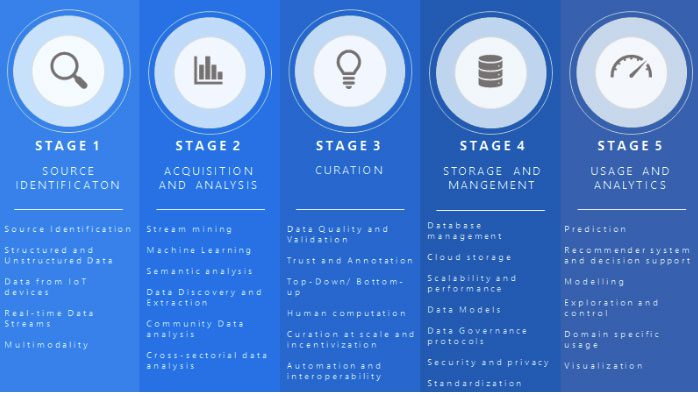
3. BENEFITS OF HAVING DATA GOVERNANCE AND ANALYTICS
By carefully selecting policies and procedures for data collection mentioned in the previous part overall value can be enhanced to a great extent. Few values driven by organizing metadata are
i. Improved data quality by
- Elimination of data duplication that will result in non-ambiguous results
- Enabling Data Consistency
- Enhanced of Data Accuracy
- Increased Data Availability
ii. Improved coordination and collaboration among the team by
- Elimination of manual data cataloging
- Emphasis on data reusability
- Accountability by Multi-level authorizations
- Increased Data Availability
iii. Improved Data Performance by
- Speedy data operations for creation, modification, replication and deletion of data
- Automated procedures- identification and classification of data
- Flexibility and scalability for data processing
- Auto-categorization: automated classification of data through Machine Learning algorithms
iv. Secured Environment for Data
- Pseudonymous usage of data as per the General Data Protection Regulation (GDPR). It’s a safeguarding policy that reduces privacy risks
- For public cloud environments, service providers like GCP, AWS, Azure provides an impressive set of security services to choose from
v. Facilitate Audit Trials
- By organizing reports on progression based on a series of events
- By maintaining operational integrity and proof of compliance
- By monitoring and validating activities
vi. Improved decision making backed by data and insights through
- ML models: Data Training to a recommender system
- Predictive modeling to enable future predictions based on historical data
- Visualization and dashboards that helps to get from a bird’s eye view of the insights to drilling down to a key source of success or failure
vii. Bigger Picture
- Less number of errors that will cost you a good amount of money
- Improved Business planning
- Improved Financial performance
4. USING ANALYTICS TO ACHIEVE BUSINESS INTELLIGENCE
Business Intelligence (BI) will become a continuous process once we have executed all the steps for data collection and analysis. Big Data empowers you to rapidly demonstrate monstrous volumes of organized and unstructured information from numerous sources. For value chain management, this can help increment visibility and give further bits of knowledge into the entire chain. Utilizing the data, value chain associations can improve your reaction to unpredictable interests or the risk, for instance, and diminish the worries identified with the current issue. It will likewise be urgent for you to develop your job from a value-based facilitator to confidant in business counselors.
Visualizing value chain metrics transforms over the immense measures of information accessible over various chain elements into effortlessly read dashboards and measurements showed progressively that administrators and planners can use to check the soundness of their operations. The outcome is quicker and increasingly effective dynamic. Here is a couple of the methods in which visualization of value chain data can push everyday activities:
- Proactive customer service
- Reaction to sudden economic changes
- Optimization for improved efficiency and results
- Real-time sourcing and cost-benefit analysis
- Identification of bottlenecks in processes
5. PRISMBERRY’S CLOUD-BASED DATA SOLUTIONS ARE INTENDED TO ASSIST BUSINESSES WITH THEIR GOALS EASILY AND PROFICIENTLY BY
- Modernizing Data and storage for all your primary activities in a value chain
- Governing your data at scale
- Developing customized solutions that will assist the primary objective
- Providing Insights through Big Data Analytics
- Offloading your on-premises workloads to the cloud
CONCLUSION
Organizations in the present pattern of worldwide guidelines and the development of the utilization of data-enabled systems should take a shot at dealing with their Big Data Entities more productively and use automation, Machine Learning, and AI to comprehend the effect of transformation on their business. They need an arrangement that scales their business procedures and causes them all through their excursion of information curation, information disclosure, quality, and administration.